The rise of generative AI
In recent years, we have witnessed the rise of generating content with large language models, which has revolutionized the way we create and consume information. These language models, powered by deep learning algorithms, have the ability to understand and generate human-like text, making them invaluable tools for various applications such as writing assistance, translation, and even storytelling.
One of the key driving forces behind the popularity of large language models is their ability to generate coherent and contextually relevant text. These models are trained on massive amounts of data, allowing them to learn the intricacies of language and its nuances. As a result, they can produce text that is indistinguishable from that written by a human. This has opened up new possibilities for content creators and writers, who can now leverage these models to generate ideas, brainstorm, and even draft entire pieces of content.
Furthermore, large language models have democratized content creation in many ways. Traditionally, producing high-quality content required expertise, time, and resources. However, with the advent of these models, anyone with access to them can generate compelling and engaging text. This has leveled the playing field, allowing individuals and businesses of all sizes to create content that resonates with their target audience.
But ...
Using large language models to generate content certainly has its flaws. One of the most significant concerns is the issue of bias. These models are trained on vast amounts of data, which means that any biases present in the training data are likely to be reflected in the generated content. This can perpetuate existing biases and stereotypes, leading to unfair or discriminatory outcomes.
Another big issue is, language models can sometimes generate inaccurate or misleading information. Since they are trained on existing data, they may not always have access to the most up-to-date or reliable information. This can result in the spread of false or outdated information, which can be detrimental, especially in fields where accuracy is crucial, such as medicine or law.
So, generative based search and reference based search
Using reference answer-based AI has become increasingly needed in various fields, ranging from customer service to education. Introducing it in BrainAtom search utilizes a delicate inferring design over disordered data to provide accurate and relevant answers to user queries. This will help you to have much more confidence in the answer as it comes from some real person and relevant to your seeking.
One of the key factors influencing the reliability of reference answer-based AI is the quality of the data source it is used for inferring. The AI system relies on correctness of the information to generate its responses. If the data used is outdated, biased, or incomplete, it can impact the reliability of the system.
Another aspect to consider is the AI’s ability to understand context and nuances. While reference answer-based AI can provide accurate answers based on the information it has been trained on, it may struggle to comprehend the subtleties of certain queries. This can lead to incorrect or incomplete responses, especially in complex or ambiguous situations. Human intervention or oversight may be necessary to ensure the reliability of the AI’s answers.
Reference Answer =
confidence
evolution of classic search
Avoid flaw in generative AI
Source and reference based answer
Decrease the time in checking
You got both: generative + reference
.
always traceable to source
This enable you to check more.
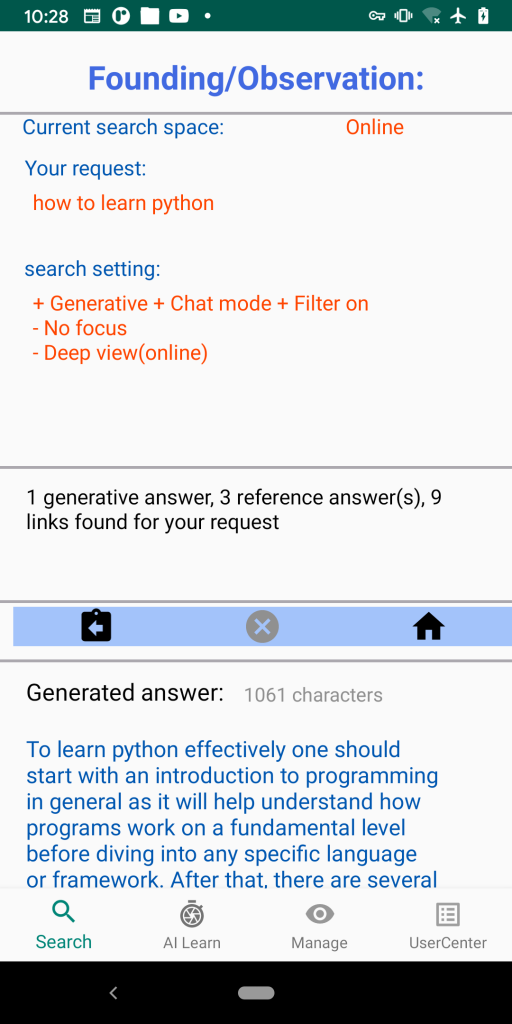