Groundbreaking design on the limit of context
The Big limitation
Large language models, such as the ones used in AI systems, have made significant advancements in natural language processing and generation. They have the ability to understand and produce human-like text, which has opened up a wide range of applications in various fields. However, these models also come with certain limitations, particularly in terms of context.
One of the main limitations of current large language models is the lack of contextual understanding. While they excel at generating coherent and grammatically correct sentences, they struggle to grasp the nuances of meaning and context. These models primarily rely on statistical patterns in the data they were trained on, which means they may not always comprehend the true intent behind a given prompt or query.
This limitation can have a profound effect on the outcomes generated by AI models. For example, when asked a complex question, a model may provide an answer that is factually correct but lacks the necessary context. This can lead to misleading or incomplete information being presented to users. Additionally, without a deep understanding of context, models may struggle to accurately interpret ambiguous statements or sarcasm, potentially resulting in erroneous or inappropriate responses.
Another challenge related to context is the issue of bias. AI models learn from vast amounts of data, including text from the internet, which can contain inherent biases and prejudices. Without the ability to fully comprehend the context, these biases can be perpetuated and amplified in the generated text. This can lead to biased or discriminatory outputs that reflect societal prejudices rather than providing fair and neutral information.
We did it and user could enjoy the freedom of unlimited context
Breaking the limitations of context in current large language models is a task that requires innovative approaches and creative thinking. While these models have made significant progress in understanding and generating text, they still struggle with understanding the nuances of context and generating coherent and contextually appropriate responses. To overcome this challenge, several strategies can be employed, including special system design, unique algorithms, and sophisticated strategies.
Now we are pround to say the system could handle millions of tokens as context easily. This is really a hard task that needs deep understanding of the requirements and products, so much effort has been contributed to the final implementation.
How to check that
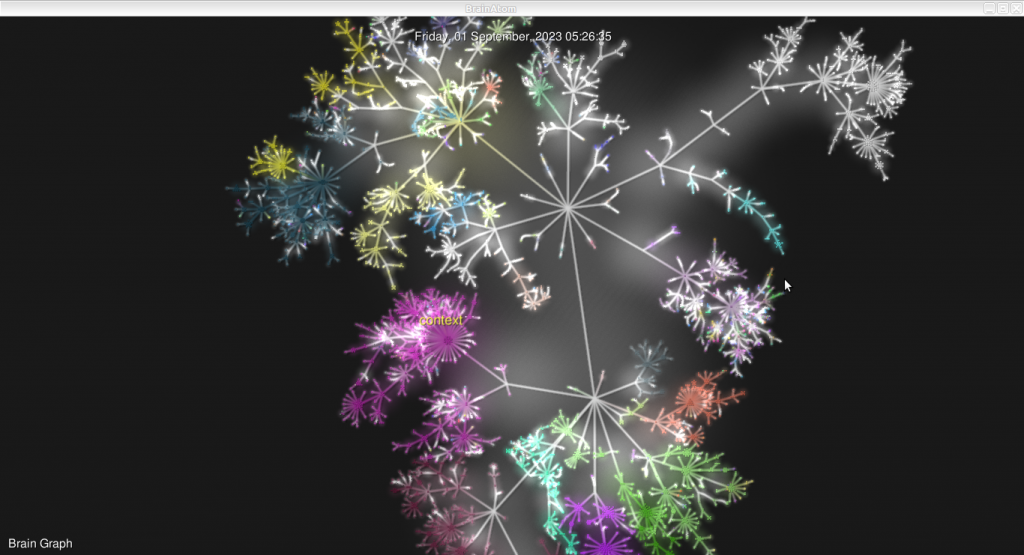
You could randomly find a book and ask the Finch to learn it for you. Repeat that step for X times. Then you could ask or search with paramind AI, all the results will be shown before you!
We usually run our AI on knowledge graph shown above. It provides what we need in real time and helps us a lot, especially there are so much local data that LLM can not provide to you.